
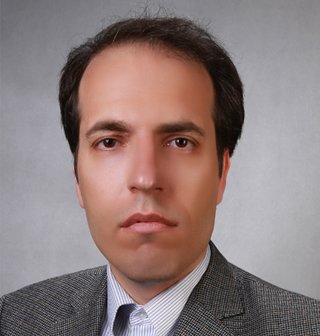
Dr. Mohammad Ali Abam
Software Group
Ph.D. in Computer Science, Eindhoven University of Technology, 2007
Computational Geometry
Massive Data Algorithms
Randomized Algorithms

Dr. Morteza Amini
Network and Security Group
Ph.D. in Computer Software Engineering , Sharif University of Technology, 2010
Database Security
Access Control
Intrusion Detection and Alert Correlation
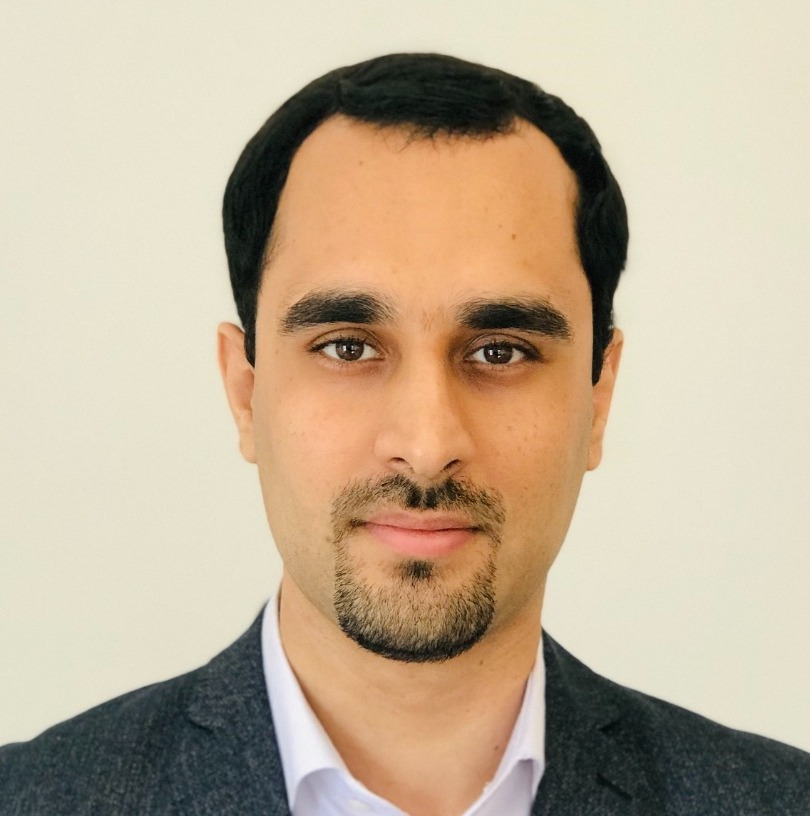
Dr.Mohsen Ansari
Computer Architecture Group
Ph.D. in Computer Engineering, Sharif University of Technology, 2021
Cyber-Physical Systems
Machine Learning for CPSs and Sensor Information Processing
Embedded Machine Learning
Edge, Fog, and Cloud Computing
Energy-Efficient, Fault-Tolerant, and Secure Computing
Thermal and Low Power Design of CPSs

Dr. Hossein Asadi
Computer Architecture Group
Ph.D. in Computer Engineering, Northeastern University, 2007
I/O & Data Storage Systems
Operating Systems
Virtualization & Cloud Computing
Dependable and Reconfigurable Computing
High-Performance Computing

Dr. Siavash Bayat-Sarmadi
Computer Architecture Group
Ph.D. in Computer Hardware, University of Waterloo, 2007
Hardware Security and Trust
Cryptographic Engineering
IOT/CPS Security

Dr. Hamid Beigy
Artificial Intelligence Group
Ph.D. in Computer Engineering, Amirkabir University of Technology, 2004
Theoritical machine learning
Data stream mining
Information retrieval
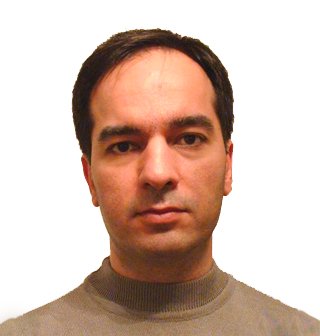
Dr. Alireza Ejlali
Computer Architecture Group
Ph.D. in Computer Engineering, Sharif University of Technology, 2006
Low Power Design
Dependable Embedded Systems
Real-Time Embedded Systems

Dr. MohammadAmin Fazli
Artificial Intelligence Group
Ph.D. in Computer Engineering, Sharif University of Technology, 2015
Software Engineering
Complex Networks
Computational Social and Economic Systems

Dr. Gholamreza Ghassem-Sani
Artificial Intelligence Group
Ph.D. in Computer Science, University of Essex, 1992
Natural Language Processing
Artificial Intelligence Planning
Multi-Agent Systems
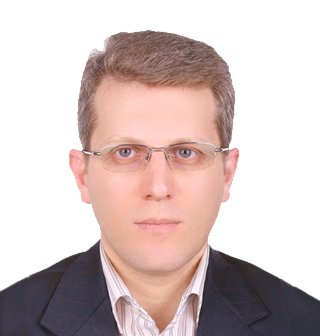
Dr. Maziar Goudarzi
Computer Architecture Group
Ph.D. in Computer Engineering, Sharif University of Technology, 2005
Warehouse-Scale Computing (cloud
big data
bioinformatics)
Hardware-Software Co-design
Green Computing and IT Infrastructure

Dr. Jafar Habibi
Software Group
Ph.D. in Computer Science, University of Manchester, 1998
Simulation
Software Engineering
Information Systems
System Analysis and Design

Dr. Shaahin Hessabi
Computer Architecture Group
Ph.D. in Electrical and Computer Engineering, University of Waterloo, 1995
Systems and Networks on Chip (SoC and NoC)
Optical Networks for Data Centers
Cyber Physical Systems
Reconfigurable and Heterogeneous Architectures
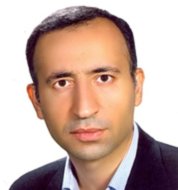
Dr. Abbas Heydarnoori
Software Group
Postdoctoral Fellow, University of Lugano, 2010
Ph.D. in Computer Science, University of Waterloo, 2009
Software Analytics
Intelligent Software Engineering
Mining Software Repositories
Software Maintenance and Evolution
Empirical Software Engineering
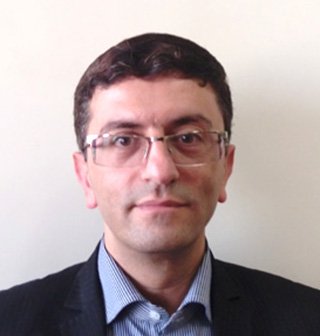
Dr. Mohammad Izadi
Software Group
Ph.D. in Computer Science, Leiden University, 2011
Ph.D. in Computer Engineering, Sharif University of Technology, 2008
Distributed and Multiagent Systems
Software and Systems Verification
Logic in Computer Science and AI
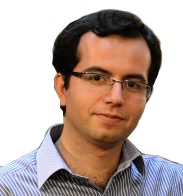
Dr. Mahdi Jafari Siavoshani
Artificial Intelligence Group
Postdoctoral Fellow, The Chinese University of Hong Kong, 2013
Ph.D. in Computer and Communication Sciences, Ecole Polytechnique Federale de Lausanne, 2012
Information Processing
Large Scale Networks
Machine Learning
Information Theory
Graphical Models
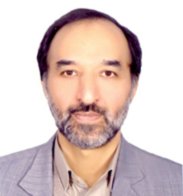
Dr. Amir Hossein Jahangir
Computer Architecture Group
Ph.D. in Industrial Informatics, National Institute of Applied Sciences, 1989
High performance Computer Architecture
Computer Network equipment test and evaluation
Hardware security
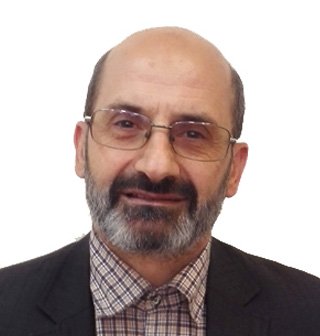
Dr. Rasool Jalili
Network and Security Group
Ph.D. in Computer Science , The University of Sydney, 1995
Operating Systems
Distributed Systems
Computer Networks
Database Systems
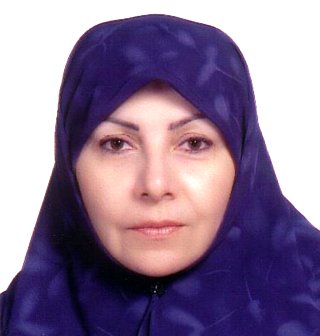
Dr. Shohreh Kasaei
Artificial Intelligence Group
Ph.D. in Electrical Engineering , Queensland University of Technology, 1998
Image Processing
Video Processing
3D Computer Vision
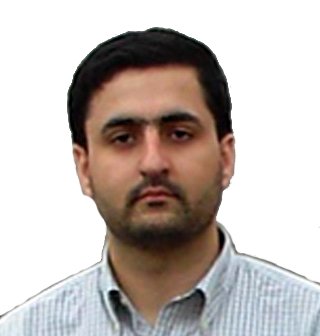
Dr. Mahdi Kharrazi
Network and Security Group
Ph.D. in Electrical Engineering , Polytechnic University, 2006
Computer Networks
Operating Systems
Computer Security
Network Security
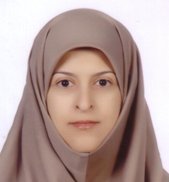
Dr. Somayyeh Koohi
Computer Architecture Group
Ph.D. in Computer Architecture , Sharif University of Technology, 2012
Bio-Photonic
Bio-Informatics
Optical Data Processing
Optical Communication
Silicon Photonics
AI Hardware Acceleration

Dr. Mohammad Taghi Manzuri
Artificial Intelligence Group
Ph.D. in Electrical and Computer Engineering , Vienna University of Technology, 1996
Signal processing
Image and Video Processing
Ambient Intelligence

Dr. Seyed-Hassan Mirian-Hosseinabadi
Software Group
Ph.D. in Computer Science, University of Essex, 1997
Formal Methods
Soft Eng.
Inf. Retrieval Systems
Data Base
Constructive Mathematics
Type Theory
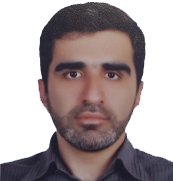
Dr. Seyed Abolfazl Motahari
Artificial Intelligence Group
Postdoctoral Fellow, University of California, 2013
Postdoctoral Fellow, University of Waterloo, 2010
Ph.D. in Electrical and Computer Engineering, University of Waterloo, 2009
Bioinformatics
Big Data Analysis
Theoretical Machine Learning
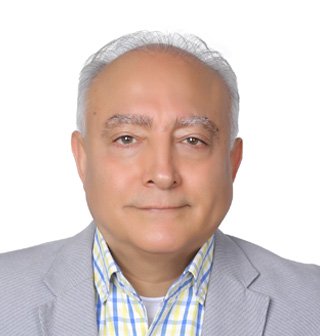
Dr. Ali Movaghar
Software Group
Ph.D. in Computer, Information and Control Engineering, University of Michigan, 1985
Performance and Dependability Modeling
Formal Verification
Cyber-Physical Systems
Distributed Real-Time Systems
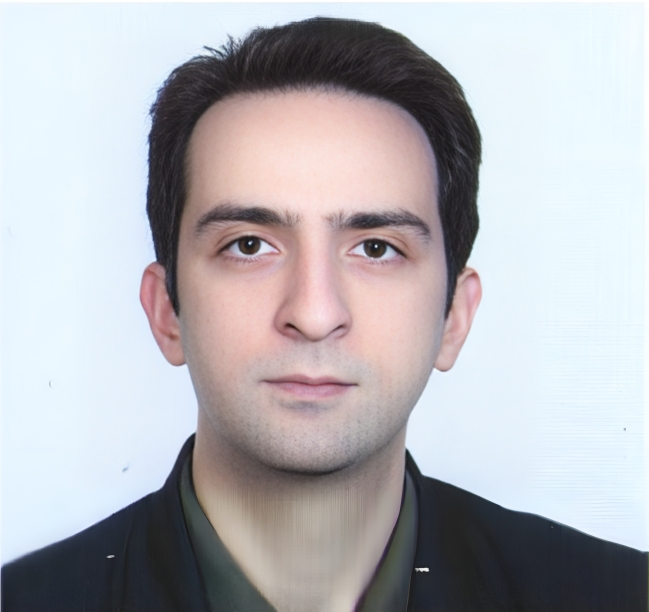
Dr. Amir Najafi
Artificial Intelligence Group
Ph.D. in Artificial Intelligence, Sharif University of Technology 2021
- Room 820
- +9821 6616 6681
- amir.najafi [at] sharif . edu
Learning Theory
Statistics
Natural Language Processing
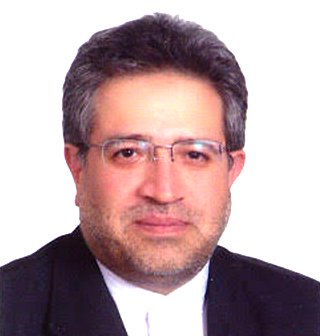
Dr. Hamid Reza Rabiee
Artificial Intelligence Group
Ph.D. in Electrical and Computer Engineering, Purdue University, 1996
Machine Learning/Big Data Analythics
Complex/Multimedia Networks
Bioinformatics
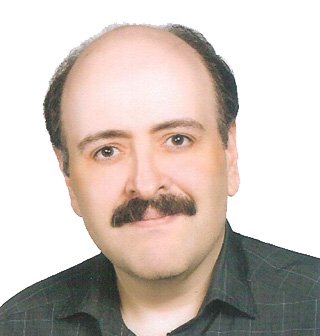
Dr. Raman Ramsin
Software Group
Ph.D. in Computer Science, University of York, 2006
Situational Method Engineering
Agile Software Development
Model-Driven Engineering

Dr. Mohammad Hossein Rohban
Artificial Intelligence Group
Ph.D. in Computer Engineering, Sharif University of Technology, 2012
Biomedical Image Analysis
Computer Vision
Machine/Deep Learning
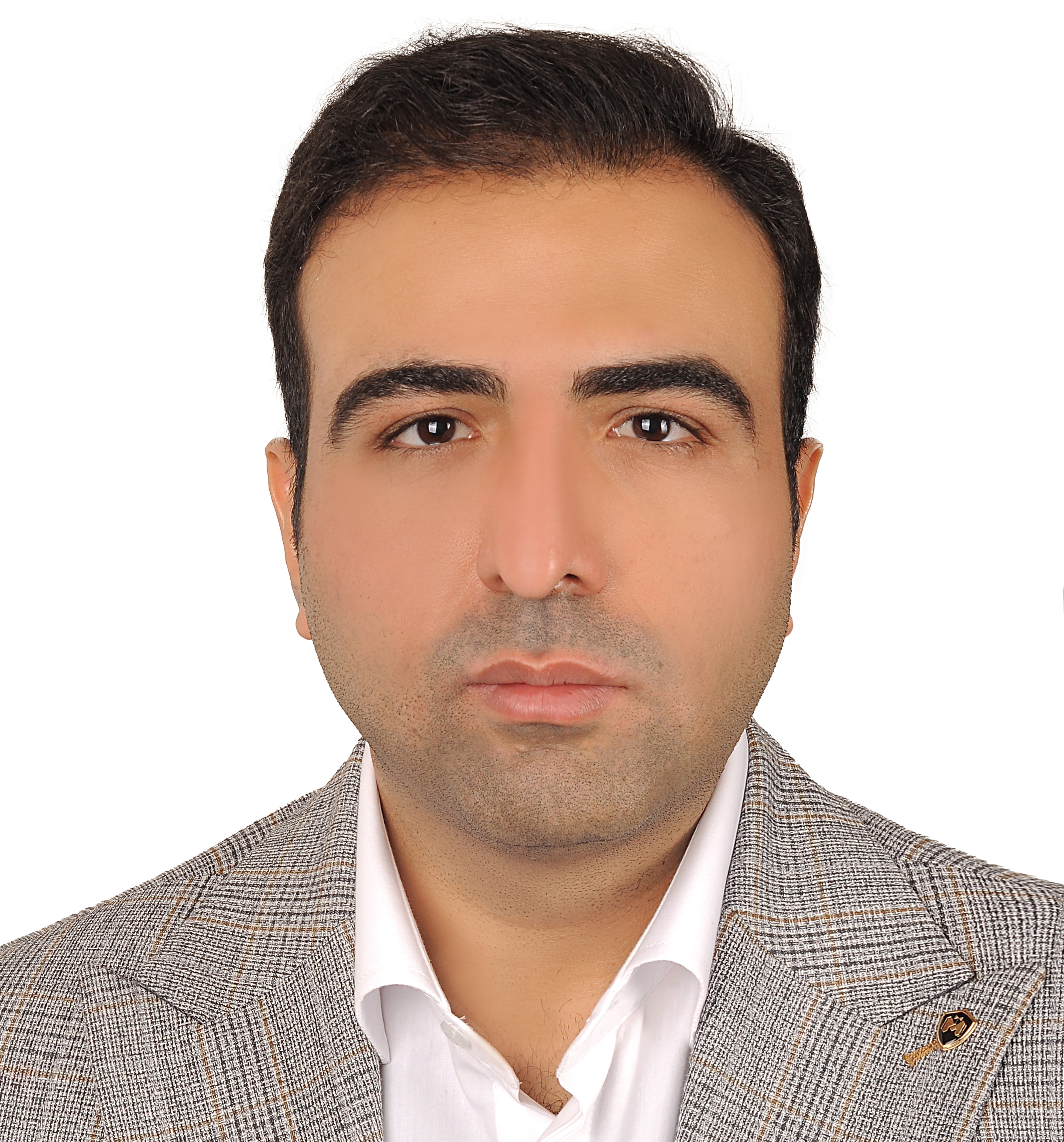
Dr. Seyed Amir Mahdi Sadeghzadeh
Network and Security Group
Ph.D. in Computer Engineering, Sharif University of Technology, 2022
Machine Learning Security
Machine Learning Privacy
Machine Learning Applications in Cybersecurity
Network Security
Blockchain
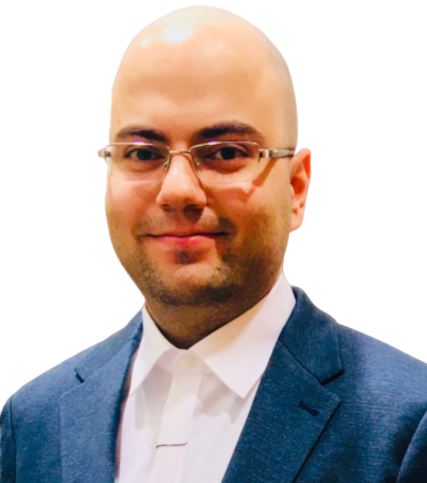
Dr. Bardia Safaei
Network and Security Group
Ph.D. in Computer Engineering, Sharif University of Technology, 2021
Internet of Things (IoT)
Wireless Sensor Networks (WSN)
Mobile Ad-hoc Networks (MANET)
Edge/Fog/Cloud Computing
Vehicular Ad-hoc Networks (VANET)
Embedded Systems
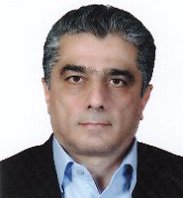
Dr. Hossein Sameti
Artificial Intelligence Group
Ph.D. in Electrical Engineering, University of Waterloo, 1995
Speech and Language Modeling
Speech Recognition and Synthesis
Speaker Identification and Verification
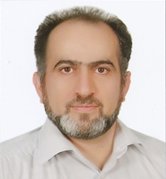
Dr. Hamid Sarbazi-Azad
Computer Architecture Group
Ph.D. in Computer Science, University of Glasgow, 2002
Advanced computer architectures
Memory/storage systems
Multi- and many-core systems
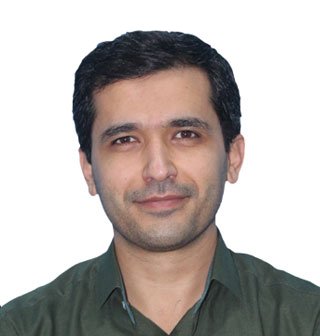
Dr. Ali Sharifi-Zarchi
Artificial Intelligence Group
Ph.D. in Bioinformatics, Institute of Biophysics & Biochemistry, University of Tehran, 2015
Algorithm Methods in Bio-informattion.
Deep-learning for Computational Biology
Bio-medical Data Analysis for Precision Medicine
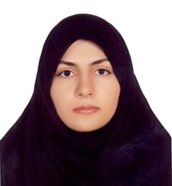
Dr. Mahdieh Soleymani Baghshah
Artificial Intelligence Group
Ph.D. in Computer Engineering, Sharif University of Technology, 2010
Machine Learning
Deep Learning
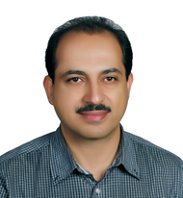
Dr. Hamid Zarrabi-Zadeh
Software Group
Ph.D. in Computer Science, University of Waterloo, 2008
Algorithms
Computational Geometry
Algorithmic Graph Theory